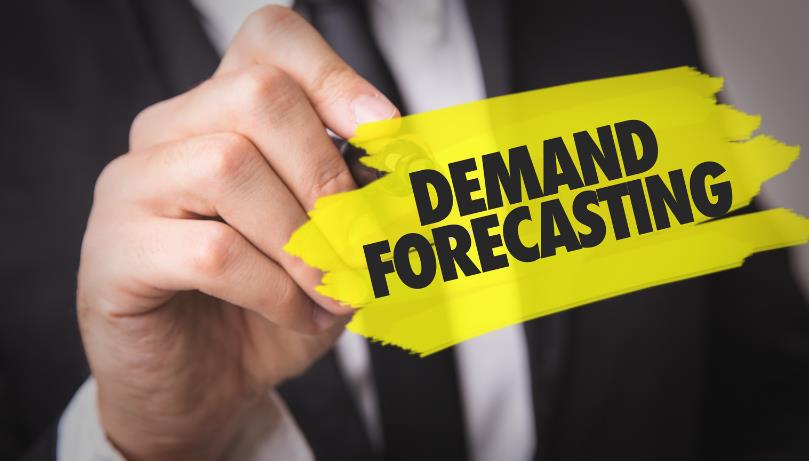
The Role of Machine Learning in Demand Forecasting for Businesses
Demand forecasting plays a crucial role in any business. It helps businesses make better decisions about the future and prepare for it by having an accurate prediction of their demand. When it comes to forecasting, machine learning has emerged as one of the most important tools businesses can use. In this post, we’ll explain how machine learning works in demand forecasting and why it’s so useful for businesses.
The Importance of Accurate Demand Forecasting
Demand forecasting is a critical component of supply chain management. It helps businesses to plan and manage their resources effectively, saving money and increasing revenue.
It’s important to understand that demand forecasting is not an exact science; it’s an art. The key is knowing how to use data from various sources to create an accurate forecast for the future. In this article, we will discuss how machine learning can be used for this purpose as well as some other benefits of using machine-generated forecasts instead of manual ones or relying solely on historical data (which may not always be relevant).
Machine Learning in Demand Forecasting
Machine learning is the process of training a computer to learn from data, offering versatile applications such as predicting future trends and optimizing business processes. One prominent application of machine learning is demand forecasting, a critical aspect for businesses aiming to accurately meet customer demands.
By leveraging machine learning algorithms, businesses can enhance their ability to predict future trends, optimize various aspects of their operations, and ultimately improve the overall customer experience. Explore the impactful role of machine learning in demand forecasting and its diverse applications at ml demand forecasting.
Traditional vs. Machine Learning-Based Demand Forecasting
Traditional forecasting methods are based on historical data. This approach can be useful if you have enough time to collect and analyze the data, but it’s often not enough to provide accurate forecasts. Machine learning-based demand forecasting uses historical data to train a model, then applies that model to future data for more accurate predictions.
Machine learning is also more scalable than traditional forecasting methods because it doesn’t require manual effort or extensive training; instead of having an expert manually input a forecast each month or quarter, you can use machine learning algorithms that automatically update their own forecasts over time as new information becomes available (or when conditions change).
Types of Machine Learning Models for Demand Forecasting
There are four major types of machine learning models for demand forecasting:
- Supervised Learning – This type of supervised machine learning uses historical data to predict future results based on past performance. The model learns from past events, makes predictions about future events, and then compares them with actual results. If the error between predicted and actual values is high, more training data is used to improve accuracy.
- Unsupervised Learning – In contrast, unsupervised machine learning doesn’t require labeled data and works without being told what type of information to learn from its input, such as images. It discovers patterns in an unlabeled dataset autonomously through algorithms like k-means clustering or density estimation techniques like Gaussian mixture models (GMMs). For example, if you want to identify different types of objects in an image without knowing what these objects might look like beforehand (e.g., faces), it’s considered unsupervised classification. Once labeled training sets become available, they can enhance accuracy over time and reduce false positives while increasing true positives.
Benefits of Machine Learning in Demand Forecasting
A business can benefit from the use of machine learning in demand forecasting in a number of ways. First, it reduces the risk of over- or under-supplying inventory by providing accurate forecasts. This helps companies manage their inventory costs more effectively, as well as ensuring that they meet customer needs and expectations. In addition, it improves customer satisfaction because customers are receiving products on time or even earlier than expected! Businesses can also benefit from improved time to market when using machine learning to forecast demand for new products or services before they launch into production.
This allows companies to reduce their time to market and increase efficiency. Machine learning can also improve forecasting accuracy by using data from previous demand patterns as well as other relevant data sources such as weather, economic trends and macroeconomic factors. It can also adjust forecasts based on new information that becomes available over time.
Machine learning is a powerful tool that can help businesses improve their demand forecasting. It offers many benefits over traditional methods, including improved accuracy and faster results. However, machine learning is not without its drawbacks and should be used with caution before committing to it as your primary forecasting method.
Author Profile
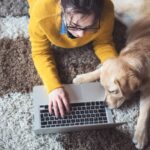
- Blogger by Passion | Contributor to many Business and Marketing Blogs in the United Kingdom | Fascinated with SEO and digital marketing and latest tech innovations |
Latest entries
Social MediaDecember 18, 2024Instagram Manager: The Key to Streamlined Social Media Success
TechnologyDecember 18, 2024Is Your Website’s Online Traffic Being Hijacked? 4 Warning Signs to Look Out for
marketingDecember 9, 2024Effective Online Marketing Strategies
TechnologyDecember 9, 2024How Windows 11 Pro Boosts Productivity and Security for Modern Students and Professionals?